MIT GOV/LAB is hosting Luke Jordan, Founder and Executive Director of the civic technology organization Grassroot, as a practitioner-in-residence in 2021. The position provides partners with resources to develop new projects and space to reflect on and share their experiences in the field, which will enable MIT GOV/LAB to better ground its research in practice.
Grassroot and MIT GOV/LAB collaborated on a distanced and low-tech leadership development course taught on the messaging platform WhatsApp and piloted with community organizers in South Africa.
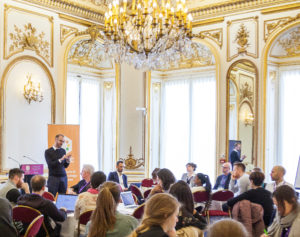
Luke Jordan presenting at TICTeC in 2019.
As a practitioner-in-residence, Jordan will be finishing a guide for practitioners on building civic technology that enables people to engage and affect their government. He’s also writing a white paper on different ways artificial intelligence and machine learning (AI/ML) can advance democracy, especially in the developing world, where Jordan says the future of democracy will be built. This paper will inform his next tech project.
Jordan spoke with MIT GOV/LAB science writer Will Sullivan about the advice in his guide, how AI/ML can advance democracy, and what he’s excited about in the coming year. The following conversation has been edited for length and clarity.
Will: So I’ve had a chance to learn about your work with Grassroot and the WhatsApp course Grassroot piloted with MIT GOV/LAB. Tell me more about what’s going to be in this guide on building civic tech?
Luke: So the guide will cover things like, “How do you hire developers? What do you look for when you’re trying to hire a developer? How do you think about a budget? How do you select underlying technology?” But the guide is called “Don’t Build It.” Because the primary lesson is, people just shouldn’t. Hopefully people who decide, despite all the warning signs, that there’s a really compelling reason to build something will find useful knowledge there.
Will: Why shouldn’t people build this stuff?
Luke: If you try to build something physical that makes no sense, nature will tell you that you’re doing something stupid. Stuff will fall down, and it will be big enough that it will be embarrassing if it’s a bad idea. If you create a badly run medical clinic that nobody uses, there will be a penalty for that.
The thing about software is, you can build anything. When people say, “could I build an app to do this,” the answer is always “yes.” And if you create a badly built app that nobody uses, nobody cares. Now, with open-source frameworks and cloud technology, almost anything can be built pretty cheap and pretty fast.
It’s also very easy to get confused about what’s actually causing problems. A natural assumption in the field is that something isn’t working because of a blocked flow of information, and that an institution would fix the problem if they knew about it. And that assumption is wrong most times.
So because you can build anything, and there’s this seductiveness of false assumptions about causes, a bad idea has smoother routes into becoming a project than in a field with more inbuilt friction. One of the ways to prevent that from happening is just to have an inbuilt bias saying, “this is probably a bad idea.”
Will: Ok, interesting — a practical guide on civic technology that warns against building tech in the first place. I’m interested in hearing what got you down this path of studying AI/ML. It seems with Grassroot that the emphasis was on building low-tech for community organizing, and now you’re going high-tech. I’m wondering what got you interested in AI/ML, and what excites you about its potential?
Luke: I deeply believe in and I’m passionate about augmenting ordinary people’s ability to act together. And I also deeply care about helping people get to places where they know they want to go, but it’s hard for them to get there.
A lot of technology gets very hyped. The thing about AI/ML is that there’s a lot of hype that’s not justified, but there actually are emerging sets of capabilities that are really interesting and could be really powerful. I think that stripped of the unnecessary hype and deployed in thoughtful ways, the set of capabilities that are emerging from AI/ML have an enormous promise to further those two goals.
For example, we are getting close to a point where we might be able to give everybody access to text-generative models that will synthesize the law for them. It’ll be able to say, “hey, there’s a law that’s 1,800 pages. But you don’t have to read 1,800 pages, here’s a two page summary, focused on the parts your community cares about, and on the strange clauses you should be careful about.” Democracy is built on the rule of law. The rule of law is a wonderful thing, but it has this inbuilt asymmetry where you need extremely technical skills to access it and use it effectively. So that means mostly rich people, rather than poor people, can do that. If some of these text models can re-balance that, that could be really powerful.
Some of the root problems of forming collective will in a modern society are just very deep and very difficult. It’s not like fancier technology alone is going to solve those problems. It’s going to need stronger and more resonant ideas, more compelling programs, more cohesive and vigorous organizations and movements. But hopefully adding some of these new AI/ML capabilities kind of adds booster fuel.
Will: What are some other ways that AI/ML can be helpful for advancing democracy?
Luke: One is AI/ML techniques running off of what are called graphs (maps of relationships), which can enable the state and state institutions, as well as organizers, to really predict unexpected effects from the combinations of different contexts. What’s important about graph data is that it’s not just a bunch of rows in a table, it shows that something is connected to something else. So if you’re building a movement, for example, if you can put membership registers and actions taken into a graph, then you might be able to identify where connecting a group that’s particularly active to another might lead to unexpected, broader effects.
Will: Of all the things that might happen in the coming year, is there one thing you’re most itching to get into?
Luke: I like to focus on practice, so I’m excited to find a partner and start building this technology. I’ll be talking to a number of other practitioners, organizations who might find one of these ideas [in the white paper] compelling or might have a different idea. And then I’ll start working on building an actual piece of AI/ML technology with a partner and integrating it into active work somewhere.
I’m aware that I’m writing a guide called “Don’t Build It,” and at the same time, here I am doing a whole project where I build something. Well, I need to find a project that ticks the boxes of, “okay, there’s a compelling reason why this actually should be built.”
But I think what will be the most significant part is a fantastic MIT undergrad (Christina Warren, a senior in computer science and writing), is going to be helping me out. Part of the idea is that I myself, or MIT GOV/LAB, will not be the people who take these ideas forward. A lot of people already have ideas, and this is just sort of adding to that fire. It’s a “let a thousand flowers bloom” kind of idea.
Photo by Nastya Dulhiier on Unsplash.